ML to fix Big Data’s ROI problems
After companies successfully built Big Data platforms, they were unsatisfied with the value they could extract from it. With the abundance of computing power, they looked at Machine Learning as a potential solution.
Machine Learning is a feature and a capability at the same time. You need to deal with a business issue on top of existing tech infrastructure and build the tech infrastructure itself.
Rushing into battle without a plan
Companies freshly entering the space without a coherent strategy will struggle to make economic sense. On the surface, all Machine Learning problems look like statistical data mining and business intelligence problems. Only after multiple POCs enterprises address the infrastructure part of the problem.
But without a medium-term strategy, the costs of this infrastructure (both from the point of time, human resources and money) will appear attached to the original POC. No wonder businesses struggle to show ROI with their ML investments.
This is also the source of the famous “It took six (ten) months to deploy a model into production” statement. Of course, it will take a long time if you spend all your resources on data cleaning and training models, and only then you start to look at moving it to production. Of course, it will be expensive if you need to amortise the cost of a full-stack solution with the benefits of a single model due to the lack of a diversified strategy.
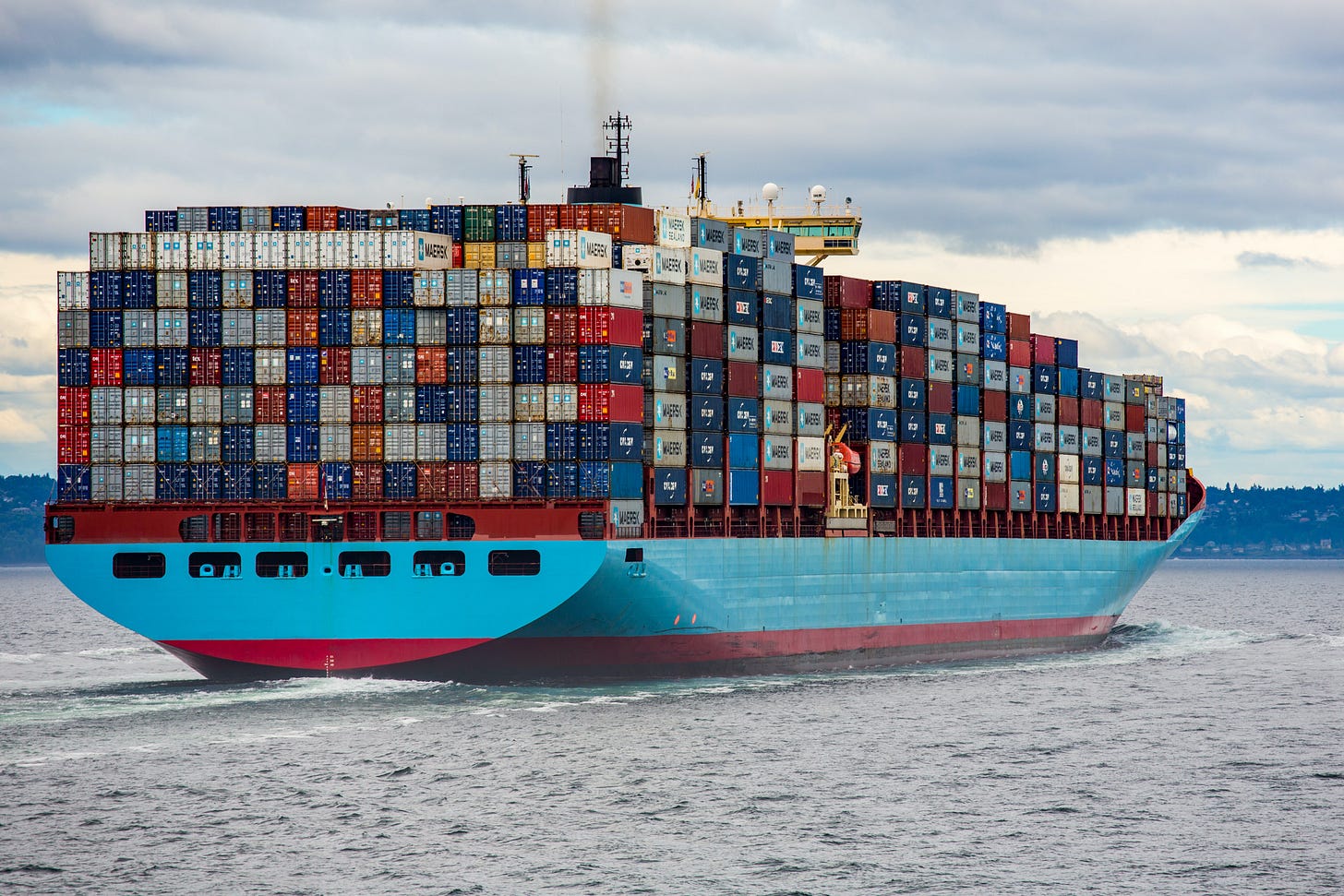
So what can you do about it?
As I said before, Machine Learning is a feature and a capability at the same time. One must separate the two and tackle them independently at the strategy level.
Gather ideas
First, look at opportunities across your business for ML features. Analyse your business for potential use cases, look at your available data sources and create a list of candidates. These will be the justification for building the capability and the infrastructure. Set one or more DS teams embedded into the business units to work on the problems and create POCs.
MVP Infrastructure
Second, establish a cross-functional working group responsible for building the infrastructure. This infrastructure should be treated as an internal product with the DSes (and other stakeholders) as users. The group’s job is to build a full-stack MVP solution and a roadmap to support the stream of POCs. Part of it is how they will move from the MVP stage to a full-scale production system when the group has more information about the requirements of successful POCs. Don’t start by making architectural decisions, or you might be building the wrong thing!
This breakdown will allow you to have a granular investment into the infrastructure and a steady stream of POCs to justify it. Once doing ML is part of business-as-usual, you can invest more into it with less risk.
As part of the Ship-30-in-30 program, I intend to write daily content on my selected topic: “Fixing Machine Learning’s productivity problem”. Subscribe to be notified in the future.