Machine learning is a new area with no standard practises or skillsets
Productionised machine learning is akin to building a plane out of parts while falling out of the sky. While also training the mechanics on how to use a torque wrench. While the torque wrench is on fire. And both of you and your boss are on fire.
Like it or not, frontier technologies are always like this. This is exactly the fun why we are in it in the first place. It is the first adopters' job to figure out best practices and disseminate them across the industry.
But this doesn't make it easy, and building teams for it are the most challenging part out of all.
Everyone is a beginner in productionised machine learning
There were barely any machine learning five years ago, and even that was at BigTech companies with their own unique circumstances. There was an exodus into the field from other areas in the last five years. Also, universities recognised the demand and started courses feeding into various jobs with a curriculum they thought to be relevant (and most importantly, can teach).
If you are working on ML at the moment, you probably worked in Business Intelligence, Data Engineering or academia before, or this is your first job straight out of university. Unfortunately, the rarest backgrounds are Software Engineering and Product Management; knowing the hiring market in these fields, this is not entirely surprising.
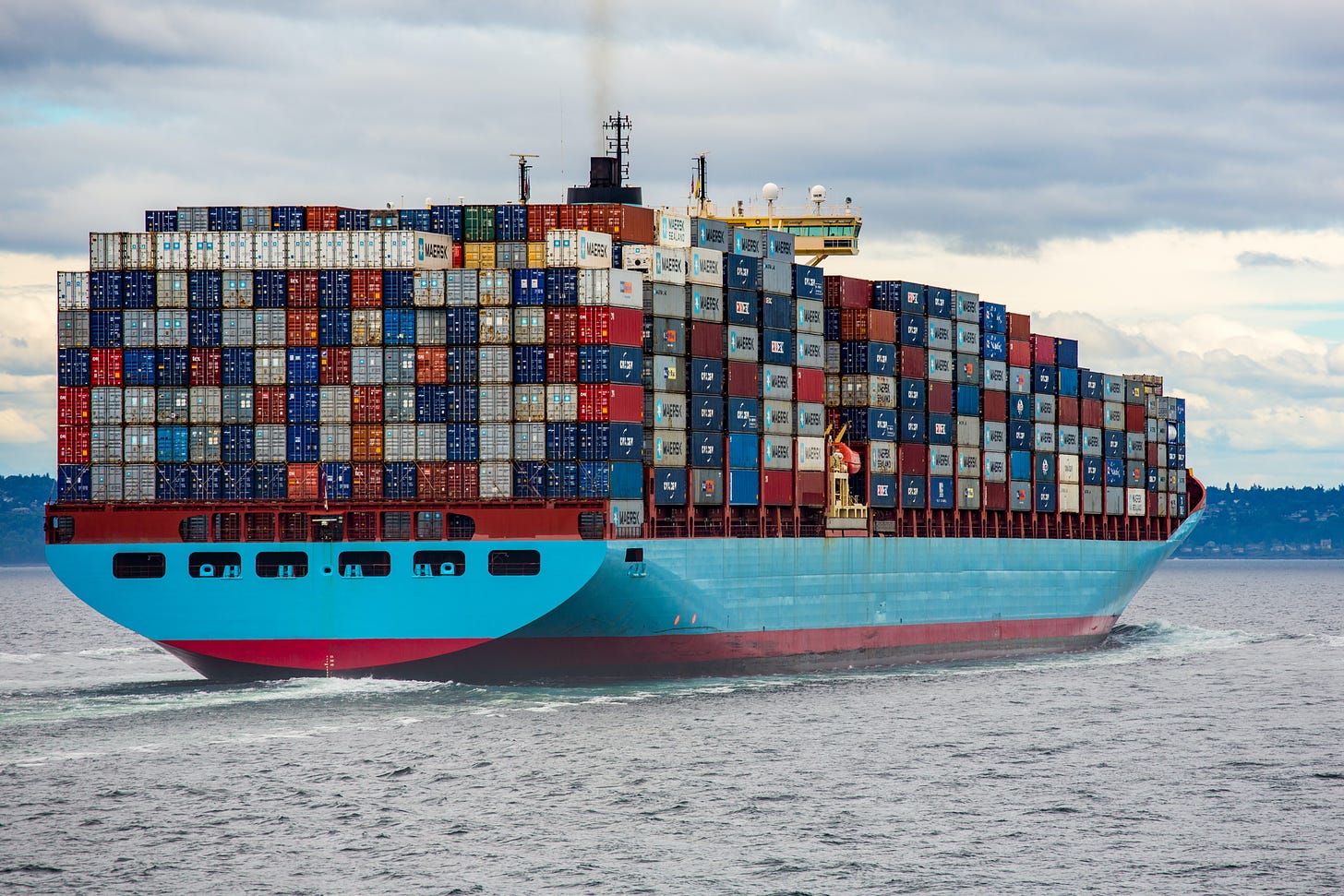
You bring your skills, but you also need to change
These quantitative jobs have their own paradigms, skills and workflows that they bring with themselves, and if they are not refactored and completed into a coherent skillset, the team will not be productive.
Business Intelligence creates one-off analysis, Data Engineering worries about data but not code, academics are excellent in theory but need help in workflow and process. And fresh graduates need to turn and complement their training with hands-on experience.
Hire people to complement your team
My advice is to start from the end result: What skillset do you need for a completed ML product? Software Engineering? Data Engineering? Analytics? Assess candidates how they complement the existing team instead of looking for polymath unicorns. (see also job adverts requiring 5 years of experience in a 2 year old product). If something is still missing (e.g. Software Engineering), reach out and find training opportunities for them. Improve yourself in Product Management and Business Operation if you came from a technical field.
And the most important one: Make sure everyone understands that they need to upskill and improve.
To quote von Clausewitz:
"You win wars with the army you have, not the army you want."
ML is a frontier technology, and everyone in it should be excited about learning new.
As part of the Ship-30-in-30 program, I intend to write daily content on my selected topic: “Fixing Machine Learning’s productivity problem”. Subscribe to be notified in the future.
If you liked to read this thread here is yesterdays about
"Causes of Machine Learning’s productivity problem: Strategy"
https://laszlo.substack.com/p/causes-of-machine-learnings-productivity